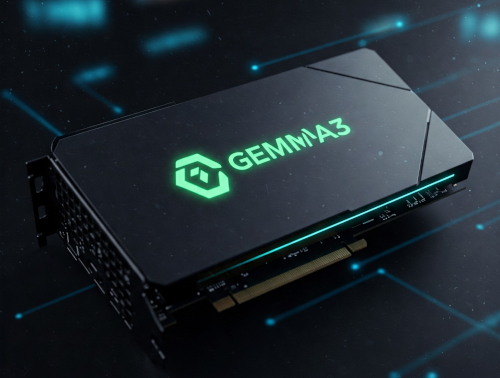
Google's recent Gemma 3 model release is definitely making waves. While the LLM hype train never stops, what really grabbed my attention wasn't just the claimed power, but the benchmarks showing Gemma 3 rivaling competitors like DeepSeek. Seeing it hold its own, especially the smaller parameter versions, felt like a real step towards more accessible AI power.
The 27B parameter version particularly caught my eye. Could my existing hardware actually handle it? Challenge accepted!
My setup is practical, not cutting-edge: a home server with a decade-old Xeon CPU, but crucially, a trusty Titan RTX GPU. It's served me well for various projects, but could this older GPU handle a modern 27B parameter LLM with reasonable performance? The appeal of running a state-of-the-art model locally, free from cloud APIs, was strong. I wanted to test the practical limits of running these new models on slightly aged consumer hardware.
So, I jumped in, setting up Gemma 3 on my Linux machine.Surprisingly, it was quite straightforward. I used Ollama, which streamlined the process significantly. After installing Ollama,launching Gemma 3 was just a single command – Ollama provides the exact one needed for your chosen model.
The result? I successfully loaded the Gemma 3 27B model using 4-bit quantization (a manageable 17GB) onto my Titan RTX with its 24GB of VRAM. A promising start – proof that even this hefty model could run within my hardware constraints!
Sat Apr 5 23:49:50 2025
+-----------------------------------------------------------------------------------------+
| NVIDIA-SMI 570.124.06 Driver Version: 570.124.06 CUDA Version: 12.8 |
|-----------------------------------------+------------------------+----------------------+
| GPU Name Persistence-M | Bus-Id Disp.A | Volatile Uncorr. ECC |
| Fan Temp Perf Pwr:Usage/Cap | Memory-Usage | GPU-Util Compute M. |
| | | MIG M. |
|=========================================+========================+======================|
| 0 NVIDIA TITAN RTX Off | 00000000:01:00.0 On | N/A |
| 41% 50C P0 273W / 280W | 20266MiB / 24576MiB | 92% Default |
| | | N/A |
+-----------------------------------------+------------------------+----------------------+
+-----------------------------------------------------------------------------------------+
| Processes: |
| GPU GI CI PID Type Process name GPU Memory |
| ID ID Usage |
|=========================================================================================|
| 0 N/A N/A 2259 G /usr/lib/xorg/Xorg 343MiB |
| 0 N/A N/A 2559 G /usr/bin/gnome-shell 91MiB |
| 0 N/A N/A 14759 G /usr/bin/nautilus 13MiB |
| 0 N/A N/A 14918 G /opt/google/chrome/chrome 3MiB |
| 0 N/A N/A 14970 G ...ersion=20250404-130110.652000 312MiB |
| 0 N/A N/A 15936 C /usr/local/bin/ollama 19464MiB |
+-----------------------------------------------------------------------------------------+
My GPU Utilization hovered around 92% during token generation, and the model was using 20 GB out of 24 GB GPU memory.
It generated around 26 tokens/s. It wasn't blazing fast, but definitely usable:
total duration: 58.239130902s
load duration: 83.277972ms
prompt eval count: 1083 token(s)
prompt eval duration: 1.36597322s
prompt eval rate: 792.84 tokens/s
eval count: 1427 token(s)
eval duration: 56.725257135s
eval rate: 25.16 tokens/s
7480be49-d298-4bd0-9dcd-23f04017226a|0|.0|96d5b379-7e1d-4dac-a6ba-1e50db561b04